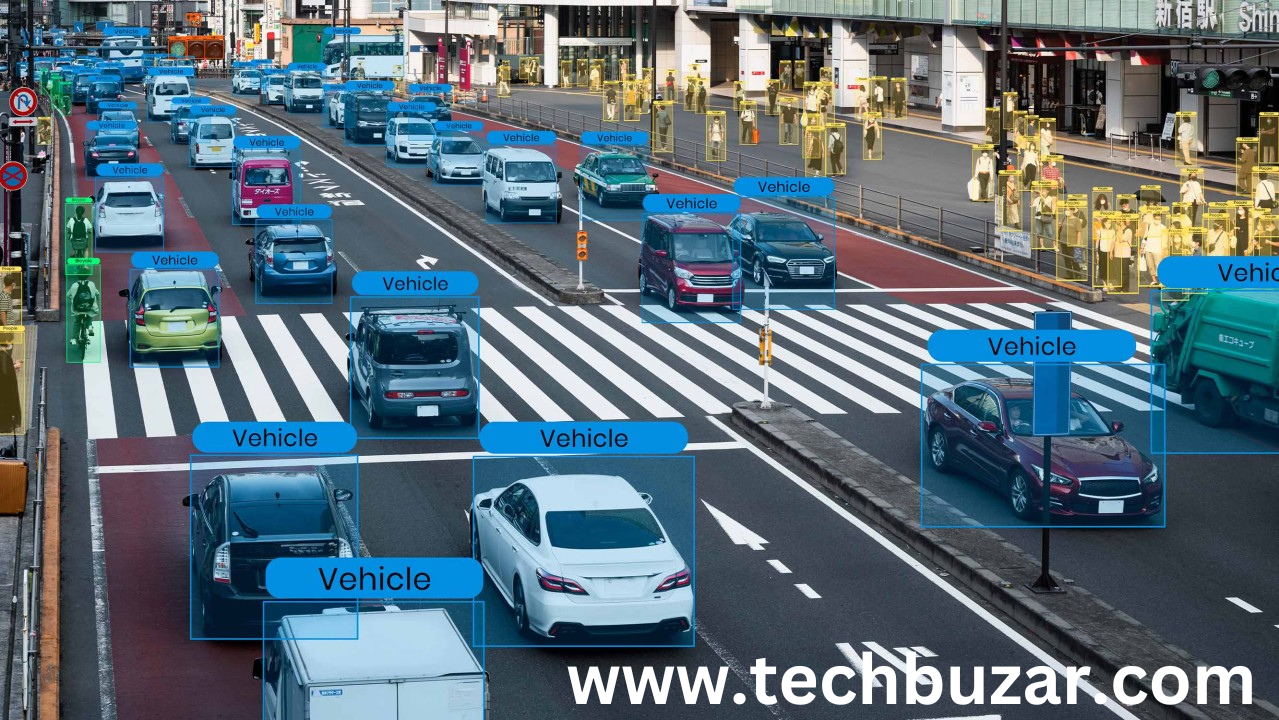
The 100% Power of Data Annotation Technology
In today’s rapidly evolving technological landscape, Data Annotation Technology plays a pivotal role in fueling the advancements of machine learning algorithms and artificial intelligence systems. As businesses and industries increasingly rely on data-driven decision-making processes, the accuracy and reliability of machine learning models become paramount. Data annotation serves as the foundation upon which these models are built, providing labeled datasets that enable machines to recognize patterns, make predictions, and automate tasks with precision.
Introduction to Data Annotation Technology
It encompasses various techniques and methodologies to annotate different types of data, including images, text, audio, and video. The annotated data serves as training data for machine learning algorithms, enabling them to learn and improve their performance over time.
Importance of Data Annotation
Enhancing Machine Learning Models
Data annotation is essential for enhancing the accuracy and efficiency of machine learning models. By providing labeled data, annotation enables algorithms to recognize patterns and make informed decisions, leading to improved performance in various applications.
Improving Accuracy and Performance
Accurate and comprehensive data annotation significantly impacts the performance of machine learning models. Well-annotated datasets ensure that algorithms can generalize effectively to unseen data, resulting in higher accuracy and reliability in real-world scenarios.
Types of Data Annotation Techniques
Data annotation techniques can be broadly categorized into three main types: manual annotation, semi-automated annotation, and automated annotation.
Manual Annotation
Manual annotation involves human annotators labeling data manually based on predefined criteria or guidelines. While this method ensures high accuracy and quality, it can be time-consuming and costly for large datasets.
Semi-Automated Annotation
Semi-automated annotation combines human expertise with machine assistance to speed up the annotation process. Human annotators review and refine annotations generated by machine algorithms, leveraging the benefits of automation while maintaining accuracy and quality.
Automated Annotation
Automated annotation utilizes machine learning algorithms to label data automatically without human intervention. While this approach offers scalability and efficiency, it may require extensive training and validation to ensure accuracy and reliability.
Commonly Annotated Data Types
Data annotation is applicable to various types of data, including images, text, audio, and video.
Image Annotation
Image annotation involves labeling objects, regions, or attributes within images to facilitate object recognition, image classification, and object detection tasks.
Text Annotation
Text annotation encompasses labeling textual data, such as documents, articles, or tweets, to extract valuable information for natural language processing (NLP) applications like sentiment analysis, named entity recognition, and text summarization.
Audio Annotation
Audio annotation involves transcribing spoken words, identifying sounds, or annotating audio signals to support speech recognition, audio classification, and sound event detection tasks.
Challenges in data annotation technology
Despite its significance, data annotation poses several challenges that need to be addressed for effective implementation.
Quality Control
Maintaining annotation quality is crucial to ensure the accuracy and reliability of machine learning models. Quality control measures, such as inter-annotator agreement and annotation guidelines, help mitigate errors and inconsistencies in labeled datasets.
Scalability
Scalability is a major concern in data annotation technology, especially for large-scale projects with massive datasets. Efficient annotation workflows, task automation, and crowd-sourcing strategies can help scale annotation efforts while controlling costs and maintaining quality.
Cost
Data annotation can be expensive, particularly for complex datasets requiring extensive human labor and expertise. Cost-effective annotation strategies, such as leveraging open-source tools, crowdsourcing platforms, and outsourcing services, can help reduce the overall annotation expenses.
Best Practices in Data Annotation
Adopting best practices is essential to ensure the effectiveness and efficiency of data annotation processes.
Clear Guidelines and Instructions
Providing clear and detailed annotation guidelines and instructions helps standardize the annotation process, reduce ambiguity, and improve annotation quality.
Quality Assurance Processes
Implementing quality assurance processes, such as regular audits, reviews, and feedback loops, helps identify and rectify annotation errors and inconsistencies, ensuring the reliability of annotated datasets.
Continuous Improvement
Promoting a culture of continuous improvement encourages annotators to refine their skills, adopt new techniques, and enhance annotation quality over time.
Tools and Platforms for Data Annotation
A wide range of tools and platforms are available to facilitate data annotation tasks, catering to diverse annotation requirements and preferences.
Labeling Tools
Labeling tools, such as LabelImg, VGG Image Annotator, and Labelbox, offer intuitive interfaces and features for annotating images, videos, and other visual data.
Annotation Management Systems
Annotation management systems, like Supervisely, Scale AI, and Label Studio, provide end-to-end solutions for managing annotation projects, collaborating with teams, and tracking annotation progress.
Applications of Data Annotation Technology
Data annotation finds applications across various industries and domains, enabling advancements in critical fields such as healthcare, automotive, and natural language processing.
Autonomous Vehicles
In the automotive industry, data annotation is essential for training autonomous vehicles to perceive and interpret their surroundings, enabling safe navigation and decision-making on the road.
Medical Imaging
In healthcare, data annotation facilitates the analysis of medical images, such as X-rays, MRIs, and CT scans, to assist in diagnosis, treatment planning, and disease monitoring.
Natural Language Processing
In NLP applications, data annotation enables the training of language models for tasks such as machine translation, text generation, and sentiment analysis, enhancing human-computer interaction and communication.
Future Trends in Data Annotation Technology
As technology continues to evolve, several emerging trends are shaping the future of data annotation technology
AI-driven Annotation
AI-driven annotation techniques, such as active learning, self-supervised learning, and weak supervision, leverage machine learning algorithms to automate and optimize the annotation process, reducing human effort and accelerating dataset creation.
Crowd Annotation
Crowd annotation platforms, like Amazon Mechanical Turk and CrowdFlower, harness the collective intelligence of crowdsourced workers to annotate large volumes of data quickly and cost-effectively, enabling scalable annotation solutions for diverse applications.
Federated Learning
Federated learning approaches enable collaborative model training across distributed datasets without centralizing sensitive data, leveraging local annotations to improve global model performance while preserving data privacy and security.
Conclusion
In conclusion, data annotation technology plays a pivotal role in advancing machine learning and artificial intelligence systems by
providing labeled datasets essential for training and refining algorithms. From image recognition to natural language processing, data annotation serves as the backbone of many AI applications, enabling machines to understand, interpret, and respond to vast amounts of data with accuracy and efficiency.
In today’s data-driven world, the importance of high-quality annotated data cannot be overstated. It not only ensures the reliability and robustness of machine learning models but also contributes to the development of innovative solutions across various industries. However, the process of data annotation comes with its own set of challenges, including maintaining quality, scalability, and cost-effectiveness.
To address these challenges, organizations must adopt best practices and leverage advanced tools and techniques for data annotation. Clear guidelines, quality assurance processes, and continuous improvement efforts are crucial for ensuring the accuracy and consistency of annotated datasets. Additionally, utilizing labeling tools, annotation management systems, and crowd annotation platforms can streamline the annotation process and enhance productivity.
Looking ahead, the future of data annotation technology holds promising opportunities for innovation and collaboration. AI-driven annotation techniques, crowd annotation platforms, and federated learning approaches are poised to revolutionize the way data is labeled and utilized for machine learning tasks. By embracing these emerging trends and advancements, organizations can unlock the full potential of data annotation technology and drive unprecedented advancements in AI-driven solutions.
In conclusion, data annotation technology serves as the cornerstone of modern AI applications, empowering machines to learn, adapt, and evolve in response to complex real-world challenges. By investing in quality data annotation practices and embracing emerging trends, organizations can harness the power of annotated data to fuel innovation, drive business growth, and shape the future of artificial intelligence.
FAQs (Frequently Asked Questions)
What is data annotation technology?
- data annotation technology involves the process of labeling raw data to make it understandable for machines, facilitating the training of machine learning algorithms.
Why is data annotation important?
- Data annotation is crucial for enhancing the accuracy and reliability of machine learning models, enabling them to recognize patterns and make informed decisions based on labeled datasets.
What are the common challenges in data annotation technology ?
- Common challenges in data annotation include maintaining quality, scalability, and cost-effectiveness, which require organizations to adopt best practices and leverage advanced tools and techniques.
How can organizations improve data annotation processes?
- Organizations can improve data annotation processes by providing clear guidelines, implementing quality assurance measures, and leveraging tools and platforms for efficient annotation workflows.
What are the future trends in data annotation technology ?
- Future trends in data annotation include AI-driven annotation techniques, crowd annotation platforms, and federated learning approaches, which are poised to revolutionize the way data is labeled and utilized for machine learning tasks.
One thought on “The 100% Power of Data Annotation Technology”